UPDATED FOR 2025
The Buying Guide for Sustainability Data Services
Introduction
For most companies, the days of voluntary sustainability reporting are over. Reporting requirements around the world are coming into effect, and sustainability teams are facing dozens of report requests each year from customers and supply chain partners. Meanwhile investors and their AI tools are scrutinizing every scrap of reported data, including detailed sustainability data. The pressure is on to produce accurate and reliable sustainability reports.
But reporting is just the start. Companies are being asked to track and report progress towards reduction goals. Detailed and reliable sustainability data is needed for the internal evaluation the business case behind reduction projects. Investors want to see spending plans in financial reporting and sustainability reporting line up.
In sum, we’re in a new era that has normalized the role of sustainability data, and upped the data quality requirements. Coarse, stale, estimated data is gone. Actual, accurate, reliable and decision-useful data is required.
This guide is written for the cross-functional teams who face this new reality and want to understand how these now standard use cases affect the nature of sustainability data, and what their options are for delivering that data. If you are in sustainability, finance, IT, legal, investor relations, accounting or procurement, this guide is for you.
The Bottom Line Upfront
Before we dig in, here are the two key takeaways. Keep these in mind as you read through the more detailed explanations of requirements and considerations.
- Automated sustainability data services save time and money. There is a compelling business case for switching from in-house semi-automated efforts to an automated data service. And there is no need to compromise, AI and and automation deliver the high-quality data you need today and in the future.
- Look for ease of use, efficient migration and seamless data flows. These features reflect the degree of automation in a system and are the key attributes that drive efficiency and cost savings. And an automated system with built-in data customization preserves your flexibility and options, as your data flows are easily adapted to changes in your other software and business systems.
Key Buying Factors
#1 Who Uses the Data?
The starting point on a buying journey is to figure out who will be using the data today, and in the future. Here GLYNT.AI’s take on this topic, based our extensive work with customers and partners.
- Reporting – Meeting regulatory requirements is table stakes. Look for data that has the specific reportable data items the EU, California, Australia, and Singapore. The superset of these requirements is likely to meet requirements everywhere. Your company may also be reporting to the Climate Disclosure Project (CDP) on a voluntary basis, if so add those fields. Your data must be audit-ready and spending on your reduction plans must be aligned with similar disclosures in your financial statements.
- Operations – While reporting may need high-level data, operations needs detailed data to identify and implement profitable savings projects. Look for extensible sustainability data systems that can do the high-level reporting and can added meter, sub-meter, asset level, and equipment level detail. Make sure the detailed data is aligned and validated to roll up to the high-level reporting, or you might find yourself with energy use from one reporting system that exceeds that reported by another. This is a surprisingly common frustration.
- Investors – Investors can make money from sustainability data, so they – and their AI tools – scrutinize it closely. Investors combine financial data, sustainability data and other data sources into a single view of a company and its future to make predictions on performance and investor returns. If your data fails this test, you’ll get the questions – and a lower company valuation. To meet investor needs, companies need accurate, reliable data, with audits, and integration of data and narratives between financial and sustainability reporting. Companies with operations in Europe should expect detailed questions from European investors, so be ready with additional layers of audited, aligned sustainability data.
- Enterprise AI – Today, sustainability is a rather siloed function and focused on acquiring automated data services to gain more accurate and reliable data at lower cost. But in the world of AI, tomorrow is coming fast. While LLMs are powerful tools, they are incredibly data hungry. Future proof your sustainability data choice by ensure that your data is accurate, verified and enriched with metatags that enable easy integration into AP, ERP, procurement and FP&A systems. Or flow your sustainability data into a central repository, such as a data lakehouse, that is accessible by enterprise AI. Either way, when you are ready for enterprise AI your data will be ready too.
Learn more about the three pillars of value for sustainability data
Sustainability Data Requirements by Use Case
#2 The Reliability Index: How to Measure “Accurate, Reliable and Decision-Useful”
Financial accounting and reporting is a well-established field, with financial regulations and audits focusing on whether reports are “accurate, reliable and decision-useful.” Suppose ACME Corp released its financial reports, and Janet Smith relied on them to make an investment. If ACME released inaccurate or incomplete data, or selective data, then Janet’s investment decision could lead to losses. Sustainability data and reporting has the same burden as financial reporting, and this is also a requirement of the GHG protocol.
To better understand the potential risk exposure of various data preparation systems, GLYNT.AI developed the Reliability Index for Sustainability Data. The key metrics are shown in the table below. Multiply the four metrics together to get the Reliability Index.
Data Quality by Type of In-House Data Preparation System
Reporting
Tracking
Audit-Ready
Finance-Grade
Expanded Reporting
Expanded Finance-Grade
Use this table and this method to score your data preparation system.
Before you match your preferred level of reliability to your use case and decide on a sustainability data system, consider the central cost tradeoff: In-house data preparation system vs automated data service.
#3 The Key Cost-Data Quality Tradeoff (It May Surprise You!)
As a leading provider of automated sustainability data services, GLYNT.AI must often demonstrate the value of our AI-powered platform. We built a TCO calculator for this purpose and have tested it extensively. Regardless of the scale of operations – from 10 sites to 1000, the following graph summarizes the key result: Automated systems deliver high quality data while saving time and money. While this story has been told many times by evangelists for automated workflows and digital transformations, it is a bit new to the sustainability team.
So as part of our TCO calculations, GLYNT.AI benchmarked the results against other metrics, such as the average cost to process business invoice data. This exercise revealed that GLYNT.AI has a highly accurate, cost-effective technology platform, with purpose-built features for sustainability data. Our powerful system delivers the results below at every scale.
And there’s a second reason that our automated data service is more efficient than in-house systems: The nature of the sustainability challenge. In-house systems require a cross-functional team to build and maintain data health: sustainability, finance, procurement, accounting, IT, governance, and data ops. Despite the fact that sustainability data has a high rate of change, requiring constant monitoring and updates to maintain data health, it is difficult to keep this group going over time. An automated data system avoids the upfront expense and the high continuing cost of the cross-functional team.
Comparison of Data Quality, Effort, and Cost: In-House Systems vs GLYNT.AI
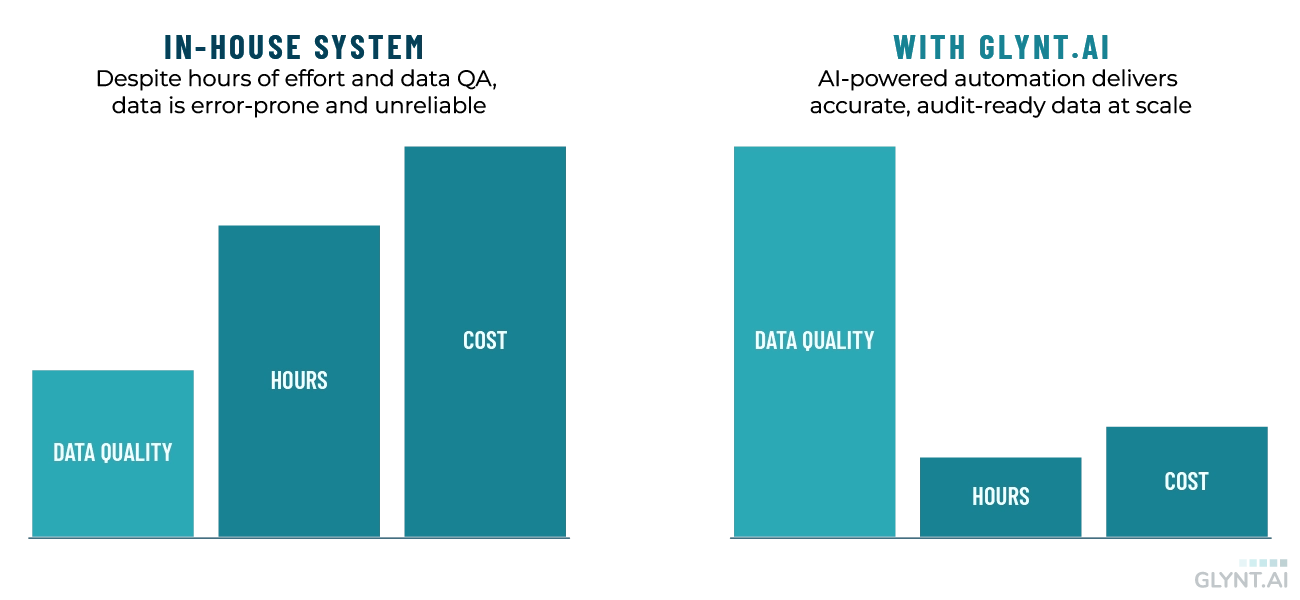
Get Ready for Field-Level Data Quality Metrics
Several industry consortia, such as Catena-X (automotive supply chain), PCAF (financial services) and the IFRS (global financial reporting standards) have laid out additional metrics on data quality that will be added to reporting standards before 2030. These standards create reportable data quality metrics, at the reported field level, and are another area to review when future-proofing a data system.
The highest quality data is direct measurement of one or more greenhouse gas from a sensor system that is audited annually for accuracy . The most frequent method for Scope 3 emissions reporting, analysis of procurement spending, has a relatively a low-quality level as spending shares are crude estimate of actual activity. As these field-level data quality standards come into place, every CFO has an overall data quality metric to report, e.g. the weighted average of the field level data quality scores. This transforms the quality of sustainability data into a personal badge of honor. Which was the point of the rating system; improvement through the avoidance of embarrassment!
Field-Level Data Quality Metrics
*Industry averages are prepared by various vendors or financial market data services to estimate emissions in investment holdings, with data fields such as emissions per dollar of value, or emissions per dollar of revenue
Sources: PCAF, IFRS, GHG Protocol and National Academy of Science
#4 Ease of Use: Identify Your Time Savings
Most large companies are reporting some type of sustainability data and have semi-automated in-house systems to collect and prepare that data, powered by hours and hours of staff time scattered across locations. These teams are fatigued and the data produced is not at the required quality levels. When looking for a new system, make sure most the hours go away. More semi-automation is not the answer!
Here are the hidden pockets of hours to look for as you review sustainability data system options:
- In-house developers. Some vendors – such as utility data providers or documents-to-data services – can provide data via an API, but a team in your company will need to integrate and map the incoming data to your software and systems.
- Setup Files Often platforms ask a customer to provide a long list of sites, account numbers and codes. This is painstaking work and can hold up an onboarding project for months. Look for systems that learn from readily available data, and don’t ask for heroic human efforts to get started.
- Customized Data As reporting standards expand, and investor data needs increase, there are an ever-expanding number of specialized metrics and data fields required for each vertical. In addition, Product Carbon Footprints are closely tied to cost accounting, always a customized data endeavor. Look for data systems with built-in customization, so your team simply informs how the data should be prepared but does not have to build the customized data flows.
- Data Lineage and Chain of Custody Reporting Sustainability data is charactered by two features: Increasing volumes of data as everyone moves to more granular data for operational wins; and constant change from changing vendors, changes in assets and so on. These reports a must-have to keep your team operating efficiently and to reduce audit costs. Without data handling tools and reporting, your team will be left building this capability in-house.
What if Sustainability Data Was Like Payroll Services?
Very few companies build their own payroll tech stack, nearly every company finds that using a third-party payroll service is simply better, faster and cheaper. Modern payroll services are experts on the employee tax regulations in every locale, with teams of subject matter experts that track and implement changes. They make it easy to on-board and off-board employees and contractors, saving hours of HR staff time. And the provide reliable and detailed reporting and data feeds finance and accounting.
What if your sustainability data service did the same? What if it was always current with local requirements, fed reliable audit-ready data into enterprise systems, had great management reporting and made it easy to on-board and off-boarding changes in vendors and asset?
This is where sustainability data services are headed, to becoming an essential and standardized function in business systems. A third-party service leverages its costs across many customers, saving you time and money; Your team to focus on what really matters. We’ve seen this story before, and it makes a lot of sense!
#5 Data System Migration: Let Technology Do the Work For You
AI-Powered Data System Migration from GLYNT.AI
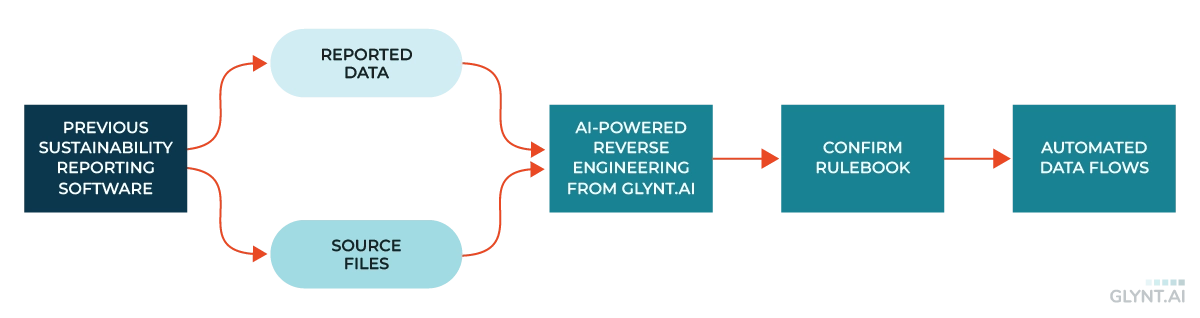
GLYNT.AI uses AI to process all types of Source Files for sustainability data, and we put that horsepower to work on migration projects. Customers give us an export of reported data from their current system and the original Source Files. Our AI will reverse engineer the data preparation process and document the rules currently used in a Rulebook. The Rulebook visually shows the data captured on the Source File, how it was transformed and reported. Once your team confirms the rules, GLYNT.AI prepares the automated data flows.
This simple migration experience helps GLYNT.AI customers change reporting software, go from ad hoc to automated data services, or both. Most customers find that Rulebook helps keep internal teams on the same page and clarifies ambiguous practices. The result is more accurate data too.
#6 Security & Compliance
As the volume of sustainability data increases, security and compliance teams get involved. With the passage of Sarbanes-Oxley (SOX) financial data has had 25 years of standards for compliance and oversight, and the multi-layered systems that are used today to keep data secure and private have matured over many years. This creates a sharp contrast with current sustainability data practices.
GLYNT.AI is SOC 1 compliant, with an annual audit. SOC 1 is the same standard that U.S. public companies must meet to report financial data under SOX. Our experience is that it takes 15 to 18 months to build in all of the tests, controls, and documentation to meet these compliance standards, and that is fairly similar to the experience of other companies implementing financial data compliance for the first time. Further, this is an on-going expense as the systems must be monitored and maintained, and staff retrained.
Companies building an in-house sustainability data system must be prepared to undergo the same expense of time and money. Companies using an automated data service should look for this type of certification. In addition, normal information security requirements, look for providers who do not monetize your data, train their AI on your data to serve others, and who use a multi-tenant, segmented system so that your data does not co-mingle with that of other customers.
Often in sustainability the word compliance is used to mean meeting regulatory reporting requirements, or the GHG Protocols. Of course your data should be prepared to be compliance with these standards. But as sustainability data systems are part of the enterprise tech stack, the data system should be compliant in the GRC sense.
When prepared at the SOC 1 level, sustainability data is inherently audit-ready, but you should look for solutions that also make audits faster and easier. Too often auditors constantly post a large number of fragmented and detailed questions to the sustainability team chasing down data preparation specifics. To cut audit expense, look for an automated data service that can help you set up audit-ready archives with data, reporting, documentation and certification. Audit-ready data is good, but audit-ready archives are needed to cut costs.
The Buyer’s Checklist
If you’re out shopping for a sustainability data system, GLYNT.AI has a simple checklist, with supporting resources.
First, review your use case. That will determine your required level of data quality and set the system requirements
Second, compare your alternatives. Use the features of the sustainability data tech stack shown to the right to evaluate functionality.
Third, crunch the numbers. Use GLYNT.AI’s TCO Calculator to compare three alternatives: in-house data systems; in-house data systems with point solutions; and automated data services.
Next Steps
Ready to see what GLYNT can do for you and your company?
Reach out to our team!
Resources
Finance-Grade Sustainability Data
Unlock Opportunities
Integrated Reporting
The TCO Calculator
Crunch Some Numbers
Contact GLYNT.AI
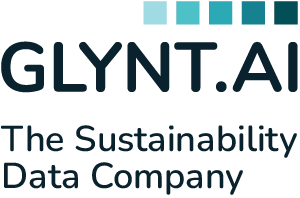
New to GLYNT.AI
© 2025 GLYNT.AI, Inc. | #betterdatafortheplanet | Terms of Use | Privacy Policy | Compliance Framework